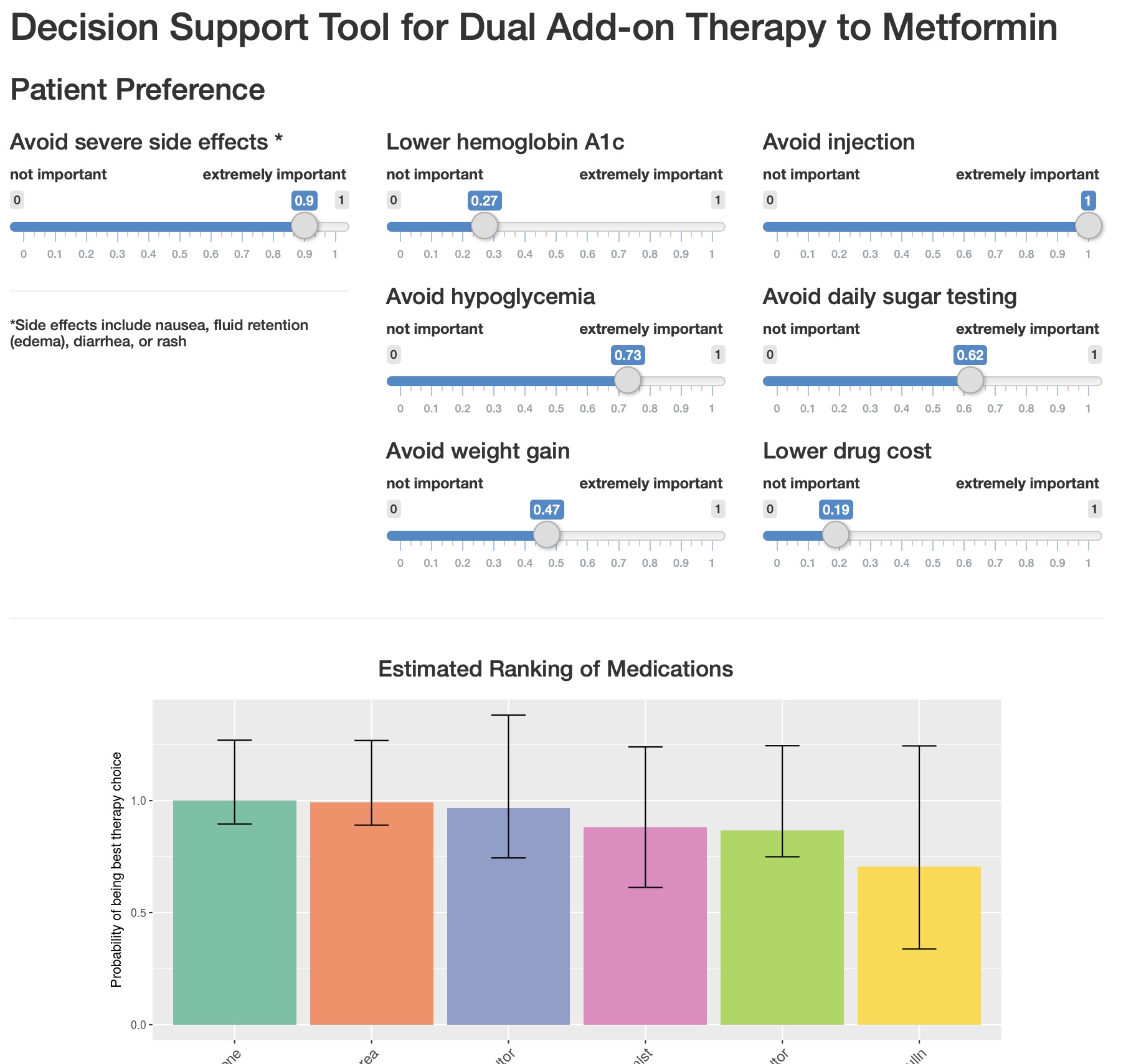
By: Waymark
Care, guided by community
We are trying to fill two key gaps in the Medicaid marketplace: (1) the need to synthesize a large amount of data from multiple disciplines (pharmacology, specialty care, psychiatry) to pick the right interventions for patients with multiple complex comorbid conditions and different preferences for treatment; and (2) the need to target interventions to the patients who would benefit most, and reduce polypharmacy among those patients who would face the most side-effects and least benefit, in the context of high demand and low supply of Medicaid providers. We provide a new workforce to serve Medicaid patients, and help that new workforce scale to address the growing population of patients insured through Medicaid (~100M people) through more efficient and more targeted interventions. We enable our care teams to focus on the highest-yield human interactions while using technology to help them know which patients to contact, when, and with what intervention. This reduces opportunity costs and helps our staff select interventions that maximize benefits and minimize risks to individual patients. Additionally, by making software that allows our staff to provide feedback on the algorithms, we fill the need to have direct provider feedback to machine learning algorithms. This feedback has been critical to identify biases in standard algorithms and address health disparities. For example, we found that the standard national algorithm for heart disease treatment systematically underestimated the risk of heart attacks for African-Americans, and were able to correct that bias [Ann Int Med, 2018, doi: 10.7326/M17-3011].